BIDSA HYBRID SEMINAR "Randomization Inference of Treatment Spillover Effects"
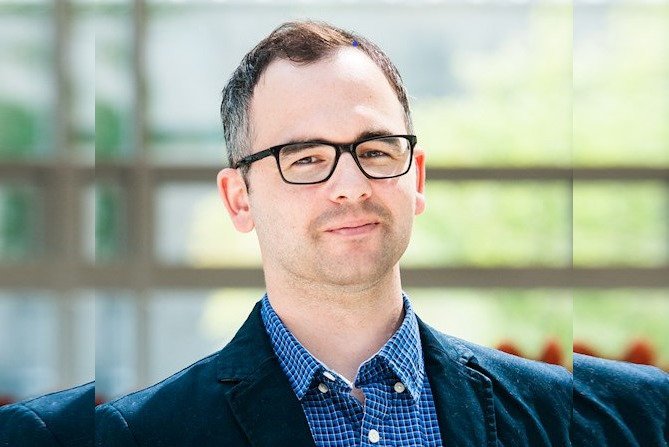
Panos Toulis, University of Chicago, Booth School of Business
Abstract:
In causal inference, interference exists when a unit’s outcome depends on the treatment assignments of other units. For example, intensive policing on one street could have a spillover effect on neighboring streets. Analyzing such effects with a model-based approach (e.g., regression) can be misleading because it requires strong, untestable assumptions on how spillovers behave asymptotically. A more robust approach is to use the randomization method, which resamples the treatment according to the experiment design in order to accurately quantify uncertainty. However, the classical method breaks down under spillovers because many null hypotheses of interest are not "sharp", i.e., they offer no information for some units in the sample. In this talk, I will propose valid, powerful, and easy-to-implement randomization tests for a general class of non-sharp null hypotheses that allow for arbitrary spillovers between units. The key idea is to represent the hypothesis of interest as a bipartite graph between units and assignments, and to find a clique of this graph. Importantly, the null hypothesis is sharp in this clique, enabling randomization-based inference conditional on the clique. I will illustrate this approach in three applications: (i) settings with clustered interference, (ii) a large-scale policing experiment in Medellín (Colombia), and (iii) an experiment designed to increase business firm performance by cultivating interfirm relationships.
How to attend online:
Join Zoom Meeting: https://unibocconi-it.zoom.us/j/93778273056
Meeting ID: 937 7827 3056
in presence: room AS01
Speaker: https://www.ptoulis.com/