BIDSA HYBRID SEMINAR "The role of Preferential Sampling in Spatial and Spatio-temporal Geostatistical Modeling"
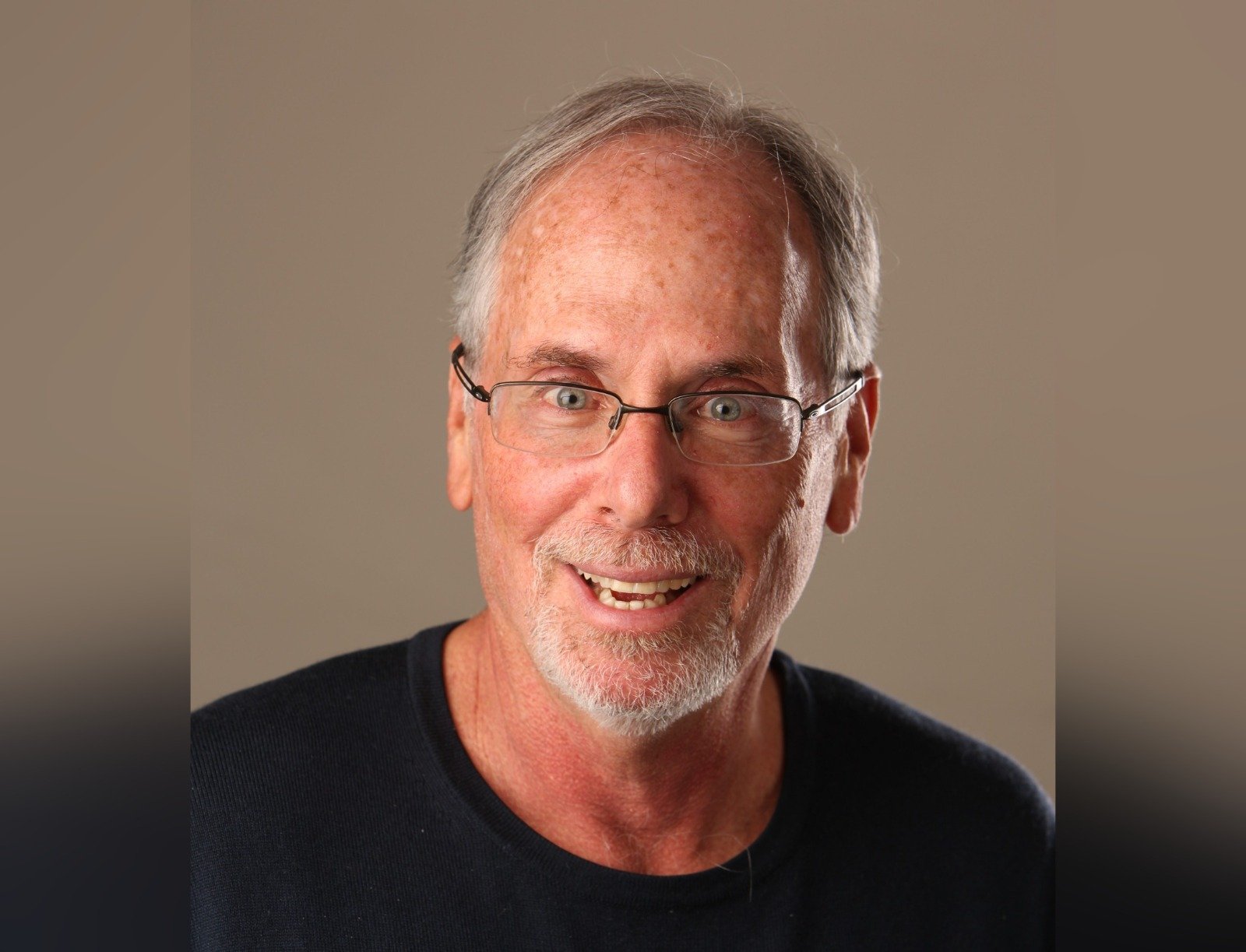
Alan Gelfand, Duke University
Abstract:
The notion of preferential sampling was introduced into the literature in the seminal paper of Diggle et al.(2010) Subsequently, there has been considerable follow up research.
A standard illustration arises in geostatistical modeling. Consider the objective of inferring about environmental exposures. If environmental monitors are only placed in locations where environmental levels tend to be high, then interpolation based upon observations from these locations will necessarily produce only high predictions. A remedy lies in suitable spatial design of the locations, e.g., a random or space-filling design for locations over the region of interest is expected to preclude such bias. However, in practice, sampling may be designed in order to learn about areas of high exposure.
While the set of sampling locations may not have been developed randomly, we study it as if it was a realization of a spatial point process. That is, it may be designed/specified in somefashion but not necessarily with the intention of being roughly uniformly distributed over D. Then, the question becomes a stochastic one: is the realization of the responses independent of the realization of the locations? If no, then we have what is called preferential sampling.
Importantly, the dependence here is stochastic dependence. Notationally/functionally, the responses are associated with the locations.
Another setting is the case of species distribution modeling with a binary response, presence or absence, recorded at locations. Here, bias can arise when sampling is designed such that ecologists will tend to sample where they expect to find individuals. This setting can be extended to data fusion where we have both presence/absence data and presence-only data. Other potential applications include missing data settings and hedonic modeling for price with property sales. Very recent work explores preferential sampling in the context of multivariate geostatistical modeling.
Fundamental issues are: (i) can we identify the occurrence of a preferential sampling effect, (ii) can we adjust inference in the presence of preferential sampling, and (iii) when can such adjustment improve predictive performance over a customary geostatistical model? We consider these issues in a modeling context and illustrate with application to presence/absence data, to property sales, and to tree data where we observe mean diameter at breast height (MDBH) and trees per hectare (TPH). (This is joint work with Shinichiro Shirota and Lucia Paci.)
How to attend online:
Join Zoom Meeting: https://unibocconi-it.zoom.us/j/96298275925
Meeting ID: 962 9827 5925
in presence: room 3-E4-SR03
Speaker: