Masked Diffusion Models for Discrete Data
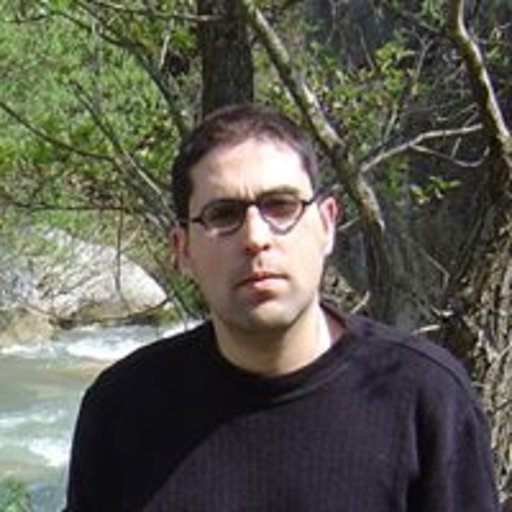
“Masked Diffusion Models for Discrete Data”
SPEAKER: Michalis Titsias (Google DeepMind)
ABSTRACT:
Masked (or absorbing) diffusion is actively explored as an alternative to autoregressive models for generative modeling of discrete data. In this work, we aim to provide a simple and general framework that unlocks the full potential of masked diffusion models. We show that the continuous-time variational objective of masked diffusion models is a simple weighted integral of cross-entropy losses. Our framework also enables training generalized masked diffusion models with state-dependent masking schedules. When evaluated by perplexity, our models trained on OpenWebText surpass prior diffusion language models at GPT-2 scale and demonstrate superior performance on zero-shot language modeling tasks. Furthermore, our models vastly outperform previous discrete diffusion models on pixel-level image modeling.