Opening the Black Box
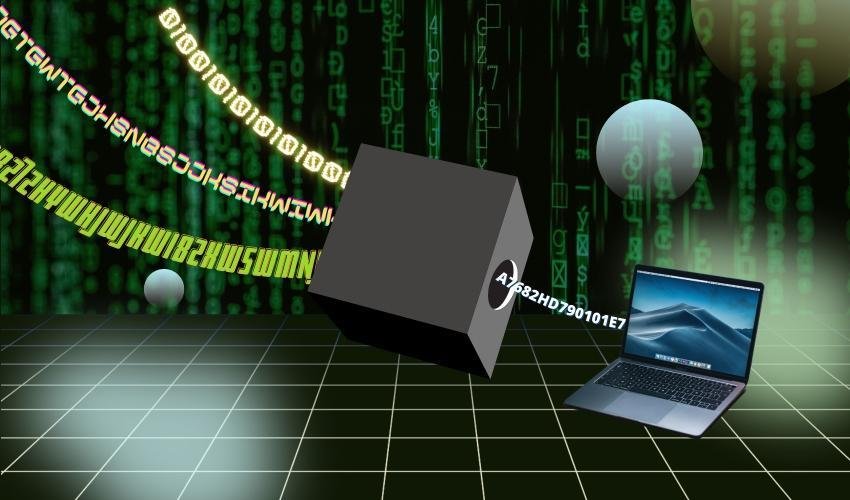
The recent hype about machine learning has been accompanied by some calls to caution from statisticians. In fact, machine learning algorithms are often described as “black boxes”, meaning that the mechanism by which their output is obtained from the input is not transparent. Moreover, such output is typically returned without any quantification of the uncertainty about it. On the contrary, transparency and uncertainty quantification are among the flagship features of statistical procedures. However, machine learning has showcased some remarkable performances (especially on big, complex and streaming data) which cannot be overlooked.
Another difference among machine learning and statistics is that machine learning focuses on prediction, while statistics is typically more interested in inferring the parameters of the assumed probabilistic model. Actually, this is an incomplete picture of statistics. In fact, the predictive approach is shared by Bayesian statistics. Named after Thomas Bayes and his celebrated theorem, this statistical approach combines prior information with data in order to provide not only “posterior” inference on the model parameters, but also effective prediction, both accompanied by a principled uncertainty quantification.
In a recent paper (see below), Sandra Fortini and Sonia Petrone, both Professors at the Bocconi Department of Decision Sciences, employed Bayesian statistics to investigate the functioning of Newton’s algorithm. The latter is a recursive procedure to classify streaming observations into different “populations” (e.g. pattern types, or signal sources), with no feedback about the correctness of previous classifications (in this sense, the classification task is said to be “unsupervised”). The success of this algorithm is due to the possibility of applying it recursively, “re-using” previous computations whenever a new observation is available. This is crucial for streaming data (data that are continuously generated). Before the work of Fortini and Petrone, it was not clear whether the efficient Newton’s algorithm was an approximation of an exact (but more computationally expensive) rigorous Bayesian procedure.
"As is often the case," explains Fortini, "this algorithm does not explicitly state a probabilistic model. However, since it relies on a predictive rule for the next observation, the tools of Bayesian statistics can be used to unveil the underlying model that is implicitly assumed. This use of the Bayesian predictive approach is not limited to Newton’s algorithm. On the contrary, it can be extended to any algorithm that relies on a predictive rule."
"This line of research," adds Petrone, "demonstrates that the Bayesian predictive approach is more than a philosophical choice. It can concretely help to shed light on algorithms whose functioning would otherwise remain obscure. And again, this is not just a scientific curiosity, because when forecasts are needed to support decisions on matters of life or death (as, for example, in the recent pandemic) we cannot trust algorithms on blind faith. Combining the speed of algorithms with the principled uncertainty quantification of Bayesian statistics can take the best of these two worlds."
Find out more
Fortini, S. and Petrone, S. (2020). “Quasi-Bayes properties of a procedure for sequential learning in mixture models.” JRSS, Series B, 82, 1087–1114. DOI: https://doi.org/10.1111/rssb.12385.
Breiman, L. (2001). “Statistical modeling: The two cultures (with comments and a rejoinder by the author).” Statistical Science, 16(3), 199-231, DOI: https://doi.org/10.1214/ss/1009213726.
by Sirio Legramanti
Source: Bocconi Knowledge