BIDSA Seminar: "Rough-glassy landscapes from inference to machine learning"
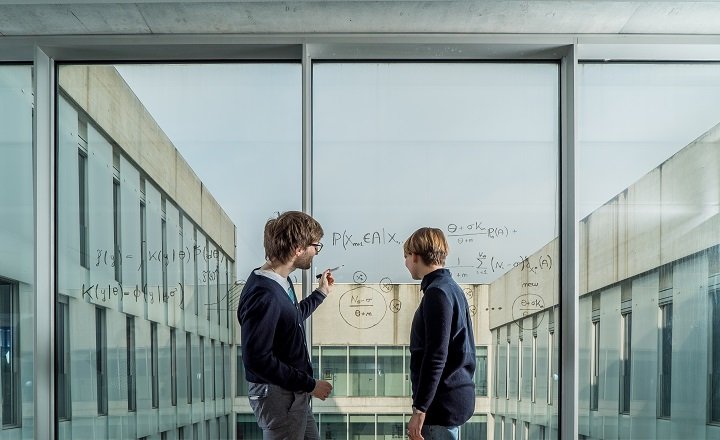
"Rough-glassy landscapes from inference to machine learning”
17 December 2018, 12:30PM
Bocconi University, Room 3.e4.sr03
via Roentgen 1, 3rd floor
ABSTRACT:
The evolution of many complex systems in physics,
biology or computer science can often be thought of as an attempt to optimize a
cost function. Such function generally depends on a highly non-linear way on
the huge number of variables parametrizing the system so that its profile
defines a high-dimensional landscape, which can be either smooth and convex, or
rugged. In this talk, the focus will be on rough cost/loss functions within the realm
of inference and machine learning. It will be first discussed the cost landscape of a
widely used inference model called spiked tensor model, hereby also
generalised, and its implications on the performances of inference algorithms.
Secondly, the speaker will report on evidences of glass-like dynamics, including aging,
during deep neural networks training, and use them to discuss the importance of
over-parametrisation, widely used in the field.
SPEAKER:
Chiara Cammarota is a Lecturer in the group of
Disordered Systems of the Mathematics Department of King’s College London. She
received her Ph.D. degree in Physics from University of Rome “Sapienza” (2010).
She worked in Paris and in Rome before moving to London in 2015. Since many
years her research is centered on the study of equilibrium and
out-of-equilibrium physics of amorphous solids via different techniques
including replica calculations, field theory approaches, Renormalization Group
studies, investigations of stochastic processes, numerical simulations. In
recent times she also started to focus on examples of disordered systems
from ecology and computer science that show strong similarities with the
physics of amorphous material.