BIDSA Webinar: "Veridical Data Science for biomedical discovery: subgroup discovery through staDISC"
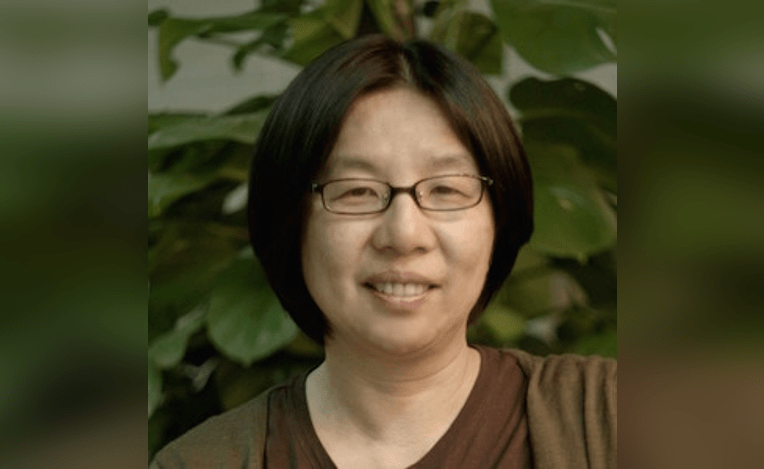
Bin Yu (UC Berkeley Statistics, EECS, CCB http:\\binyu.stat.berkeley.edu)
May 27, 2021 | 5pm CEST | ZoomAbstract:
Data Science is a pillar of A.I. and has driven most of recent cutting-edge discoveries in biomedical research. In practice, Data Science has a life cycle (DSLC) that includes problem formulation, data collection, data cleaning, modeling, result interpretation and the drawing of conclusions. Human judgement calls :wq:ware ubiquitous at every step of this process, e.g., in choosing data cleaning methods, predictive algorithms and data perturbations. Such judgment calls are often responsible for the "dangers" of A.I. To maximally mitigate these dangers, we developed a framework based on three core principles: Predictability, Computability and Stability (PCS). Through a workflow and documentation (in R Markdown or Jupyter Notebook) that allows one to manage the whole DSLC, the PCS framework unifies, streamlines and expands on the best practices of machine learning and statistics – bringing us a step forward towards veridical Data Science.
Furthermore, we will illustrate the PCS framework for Stable Discovery of Interpretable Subgroups via Calibration (StaDISC). We designed staDISC in the context of our re-analysis of the 1999-2000 VIGOR randomized clinical trial. StaDISC discovers three clinically interpretable subgroups each for the GI outcome (totaling 29.4% of the study size) and the CVT outcome (totaling 11.0%). Complementary analyses of the found subgroups using the 2001-2004 APPROVe study, a separate independently conducted RCT with 2587 patients, provides further supporting evidence for the promise of StaDISC.
Reference:
Please find below the papers that will be covered by the talk:
Speaker: