BIDSA SEMINAR SERIES "Conformal Prediction and Control"
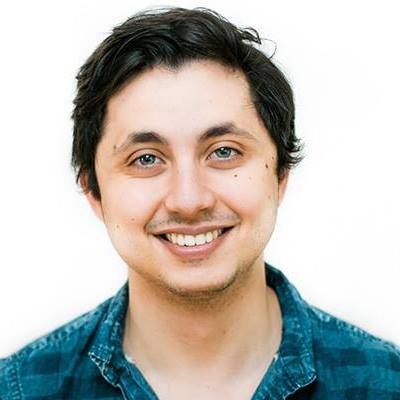
Anastasios Angelopoulos, University of California, Berkeley
Abstract:
Conformal prediction is a formally rigorous method for uncertainty quantification under the assumption that data are exchangeable, but what happens when the assumption is violated? Indeed, deployed machine-learning systems are usually exposed to ever-evolving data generating distributions for which the exchangeability assumption makes no sense. But is it possible to quantify uncertainty even under these dire conditions?
I will present an outlook on conformal prediction motivated by control theory and online optimization that addresses this question
affirmatively, providing formally rigorous uncertainty quantification even for adversarially generated data.
The seminar will be held in presence, in room 3-E4-sr03 - Roentgen Building