BIDSA SEMINAR SERIES "Optimal Transport Strikes Twice in Genomics”
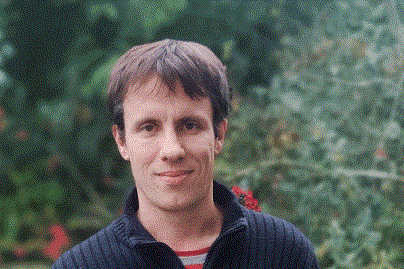
Gabriel Peyré, CNRS and Ecole Normale Supérieure
Abstract:
Optimal transport (OT) has gained a lot of interest in machine learning. It is a natural tool to compare in a geometrically faithful way probability distributions. It recently proved useful in a variety of applications to single cell genomics, in particular for two distinct but complementary types of tasks: (a) define a distance between pairs of cells over a space of genes, to perform cells clustering and multi-omics integration, (b) define a distance between two populations of cells, to perform trajectory inference in developmental biology. Vanilla OT is however plagued by several issues, which are routinely encountered in these genomics applications. This includes in particular: (i) the curse of dimensionality, since it might require a number of samples which grows exponentially with the dimension, (ii) sensitivity to outliers, since it prevents mass creation and destruction during the transport (iii) impossibility to transport between two disjoint spaces. In this talk, I will review several recent proposals to address these issues, and showcase how they work hand-in-hand to provide a comprehensive machine learning pipeline for genomics applications. The three key ingredients are: (i) entropic regularization which defines computationally efficient loss functions in high dimensions (ii) unbalanced OT, which relaxes the mass conservation to make OT robust to missing data and outliers, (iii) Gromov-Wasserstein formulation, introduced by Sturm and Memoli, which is a non-convex quadratic optimization problem defining transport between disjoint spaces (for instance genes and peaks spaces for single cell data integration). This is a joint work with Geert-Jan Huizing and Laura Cantini.
The seminar will be held in presence, in room 3-B3-sr01 - Roentgen Building
Speaker: http://www.gpeyre.com/